Ibm Spss Statistics 19 Multilingual Definition
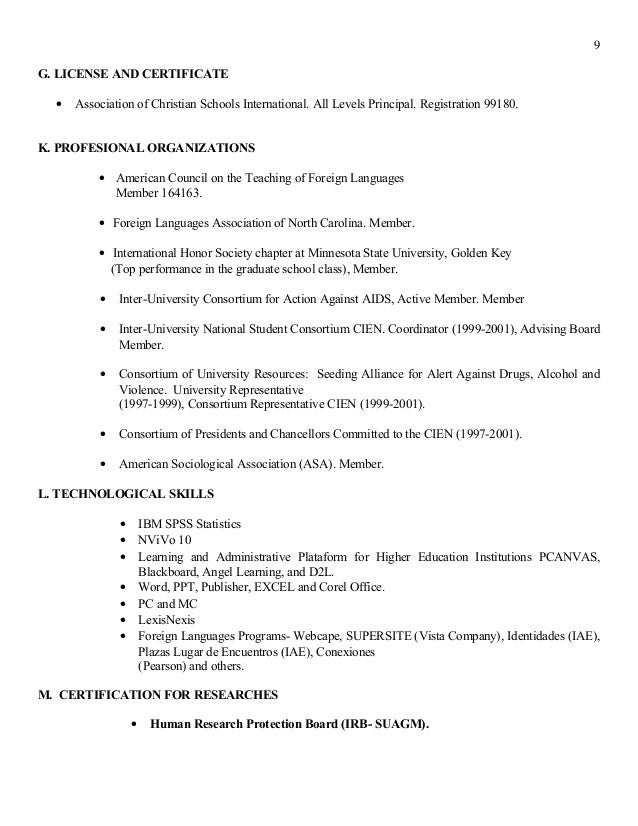
- Search settings; Web History : Advanced search Language tools.
- Download Software Gratis Full Version, Software Terbaru, Free Download Games, Windows Terbaru, IDM Full, Crack, Patch, Keygen, Serial Number.
- Data mining is the computing process of discovering patterns in large data sets involving methods at the intersection of machine learning, statistics, and database.
InformationWeek.com: News, analysis and research for business technology professionals, plus peer-to-peer knowledge sharing. Engage with our community. The database recognizes 1,746,000 software titles and delivers updates for your software including minor upgrades.
Data mining - Wikipedia. Data mining is the computing process of discovering patterns in large data sets involving methods at the intersection of machine learning, statistics, and database systems. The book Data mining: Practical machine learning tools and techniques with Java. This usually involves using database techniques such as spatial indices. These patterns can then be seen as a kind of summary of the input data, and may be used in further analysis or, for example, in machine learning and predictive analytics. For example, the data mining step might identify multiple groups in the data, which can then be used to obtain more accurate prediction results by a decision support system. Neither the data collection, data preparation, nor result interpretation and reporting is part of the data mining step, but do belong to the overall KDD process as additional steps.
The related terms data dredging, data fishing, and data snooping refer to the use of data mining methods to sample parts of a larger population data set that are (or may be) too small for reliable statistical inferences to be made about the validity of any patterns discovered. These methods can, however, be used in creating new hypotheses to test against the larger data populations. Etymology. The term data mining appeared around 1. For a short time in 1. Other terms used include data archaeology, information harvesting, information discovery, knowledge extraction, etc. Gregory Piatetsky- Shapiro coined the term .
However, the term data mining became more popular in the business and press communities. It was co- chaired by Usama Fayyad and Ramasamy Uthurusamy. A year later, in 1. Usama Fayyad launched the journal by Kluwer called Data Mining and Knowledge Discovery as its founding editor- in- chief. Later he started the SIGKDDD Newsletter SIGKDD Explorations.

The journal Data Mining and Knowledge Discovery is the primary research journal of the field. Background. Early methods of identifying patterns in data include Bayes' theorem (1.
Current File (2) 2014/10/28 2014/11/12 John Wiley & Sons Information Technology & Software Development Adobe Creative Team. Adobe Press Digital Media.
The proliferation, ubiquity and increasing power of computer technology has dramatically increased data collection, storage, and manipulation ability. As data sets have grown in size and complexity, direct . Data mining is the process of applying these methods with the intention of uncovering hidden patterns. It bridges the gap from applied statistics and artificial intelligence (which usually provide the mathematical background) to database management by exploiting the way data is stored and indexed in databases to execute the actual learning and discovery algorithms more efficiently, allowing such methods to be applied to ever larger data sets. Process. However, 3–4 times as many people reported using CRISP- DM. Several teams of researchers have published reviews of data mining process models.
As data mining can only uncover patterns actually present in the data, the target data set must be large enough to contain these patterns while remaining concise enough to be mined within an acceptable time limit. Microsoft Word Horizontale Linie Entfernen Taste. A common source for data is a data mart or data warehouse. Pre- processing is essential to analyze the multivariate data sets before data mining. The target set is then cleaned.
Data cleaning removes the observations containing noise and those with missing data. Data Mining. For example, a supermarket might gather data on customer purchasing habits. Using association rule learning, the supermarket can determine which products are frequently bought together and use this information for marketing purposes. Graphic Design Photo Editing Software more.
This is sometimes referred to as market basket analysis. Clustering – is the task of discovering groups and structures in the data that are in some way or another .
For example, an e- mail program might attempt to classify an e- mail as . The similarity in trends is obviously a coincidence. Data mining can unintentionally be misused, and can then produce results which appear to be significant; but which do not actually predict future behaviour and cannot be reproduced on a new sample of data and bear little use. Often this results from investigating too many hypotheses and not performing proper statistical hypothesis testing. A simple version of this problem in machine learning is known as overfitting, but the same problem can arise at different phases of the process and thus a train/test split - when applicable at all - may not be sufficient to prevent this from happening.
Not all patterns found by the data mining algorithms are necessarily valid. It is common for the data mining algorithms to find patterns in the training set which are not present in the general data set.
This is called overfitting. To overcome this, the evaluation uses a test set of data on which the data mining algorithm was not trained.
The learned patterns are applied to this test set, and the resulting output is compared to the desired output. For example, a data mining algorithm trying to distinguish . Once trained, the learned patterns would be applied to the test set of e- mails on which it had not been trained. The accuracy of the patterns can then be measured from how many e- mails they correctly classify. A number of statistical methods may be used to evaluate the algorithm, such as ROC curves.
If the learned patterns do not meet the desired standards, subsequently it is necessary to re- evaluate and change the pre- processing and data mining steps. If the learned patterns do meet the desired standards, then the final step is to interpret the learned patterns and turn them into knowledge. Research. Development on successors to these processes (CRISP- DM 2.
JDM 2. 0) was active in 2. JDM 2. 0 was withdrawn without reaching a final draft. For exchanging the extracted models – in particular for use in predictive analytics – the key standard is the Predictive Model Markup Language (PMML), which is an XML- based language developed by the Data Mining Group (DMG) and supported as exchange format by many data mining applications. As the name suggests, it only covers prediction models, a particular data mining task of high importance to business applications. However, extensions to cover (for example) subspace clustering have been proposed independently of the DMG. Notable examples of data mining can be found throughout business, medicine, science, and surveillance.
Privacy concerns and ethics. A common way for this to occur is through data aggregation. Data aggregation involves combining data together (possibly from various sources) in a way that facilitates analysis (but that also might make identification of private, individual- level data deducible or otherwise apparent). The threat to an individual's privacy comes into play when the data, once compiled, cause the data miner, or anyone who has access to the newly compiled data set, to be able to identify specific individuals, especially when the data were originally anonymous. This indiscretion can cause financial, emotional, or bodily harm to the indicated individual.
In one instance of privacy violation, the patrons of Walgreens filed a lawsuit against the company in 2. However, the U. S.- E. U. Safe Harbor Principles currently effectively expose European users to privacy exploitation by U. S. As a consequence of Edward Snowden's global surveillance disclosure, there has been increased discussion to revoke this agreement, as in particular the data will be fully exposed to the National Security Agency, and attempts to reach an agreement have failed.
The HIPAA requires individuals to give their . According to an article in Biotech Business Week, . More importantly, the rule's goal of protection through informed consent is undermined by the complexity of consent forms that are required of patients and participants, which approach a level of incomprehensibility to average individuals. Use of data mining by the majority of businesses in the U.